In Collections
This item can be found in the following collections:
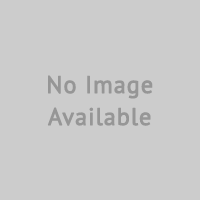
A Model-guided Method for Improving Coronary Artery Tree Extractions from CCTA images
Purpose
Automatically extracted coronary artery trees (CATs) from coronary computed tomography angiography images
could contain incorrect extractions which require manual corrections before they can be used in clinical practice.
A model-guided method for improving the extracted CAT is described to automatically detect potential incorrect
extractions and improve them.
Methods
The proposed method is a coarse-to-fine approach. A coarse improvement is first applied on all vessels in the
extracted CAT, and then a fine improvement is applied only on vessels with higher clinical significance. Based
upon a decision tree, the proposed method automatically and iteratively performs improvement operations for the
entire extracted CAT until it meets the stop criteria. The improvement of the extraction quality obtained by the
proposed method is measured using a scoring system. 18 datasets were used to determine optimal values for the<...
Show morePurpose
Automatically extracted coronary artery trees (CATs) from coronary computed tomography angiography images
could contain incorrect extractions which require manual corrections before they can be used in clinical practice.
A model-guided method for improving the extracted CAT is described to automatically detect potential incorrect
extractions and improve them.
Methods
The proposed method is a coarse-to-fine approach. A coarse improvement is first applied on all vessels in the
extracted CAT, and then a fine improvement is applied only on vessels with higher clinical significance. Based
upon a decision tree, the proposed method automatically and iteratively performs improvement operations for the
entire extracted CAT until it meets the stop criteria. The improvement of the extraction quality obtained by the
proposed method is measured using a scoring system. 18 datasets were used to determine optimal values for the
parameters involved in the model-guided method and 122 datasets were used for evaluation.
Results
Compared to the initial automatic extractions, the proposed method improves the CATs for 122 datasets from an
average quality score of 87±6 to 93±4. The developed method is able to run within 2 minutes on a typical
workstation. The difference in extraction quality after automatic improvement is negatively correlated with the
initial extraction quality (R=-0.694, P<0.001).
Conclusion
Without deteriorating the initially extracted CATs, the presented method automatically detects incorrect
extractions and improves the CATs to an average quality score of 93 guided by anatomical statistical models.
Show less- All authors
- Cao, Q.; Broersen, A.; Kitslaar, P.H.; Lelieveldt, B.P.F.; Dijkstra, J.
- Date
- 2018