In Collections
This item can be found in the following collections:
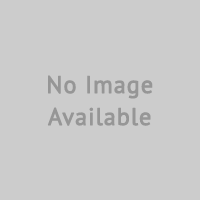
A systematic approach to analyze the computational cost of robustness in model-assisted robust optimization: a systematic approach to analyze the computational cost
Real-world optimization scenarios under uncertainty and noise are typically handled with robust optimization techniques, which re-formulate the original optimization problem into a robust counterpart, e.g., by taking an average of the function values over different perturbations to a specific input. Solving the robust counterpart instead of the original problem can significantly increase the associated computational cost, which is often overlooked in the literature to the best of our knowledge. Such an extra cost brought by robust optimization might depend on the problem landscape, the dimensionality, the severity of the uncertainty, and the formulation of the robust counterpart.
This paper targets an empirical approach that evaluates and compares the computational cost brought by different robustness formulations in Kriging-based optimization on a wide combination (300 test cases) of problems, uncertainty levels, and dimensions. We mainly focus on the CPU time taken to...
Show moreReal-world optimization scenarios under uncertainty and noise are typically handled with robust optimization techniques, which re-formulate the original optimization problem into a robust counterpart, e.g., by taking an average of the function values over different perturbations to a specific input. Solving the robust counterpart instead of the original problem can significantly increase the associated computational cost, which is often overlooked in the literature to the best of our knowledge. Such an extra cost brought by robust optimization might depend on the problem landscape, the dimensionality, the severity of the uncertainty, and the formulation of the robust counterpart.
This paper targets an empirical approach that evaluates and compares the computational cost brought by different robustness formulations in Kriging-based optimization on a wide combination (300 test cases) of problems, uncertainty levels, and dimensions. We mainly focus on the CPU time taken to find robust solutions, and choose five commonly-applied robustness formulations: `"mini-max robustness'', "mini-max regret robustness'', "expectation-based robustness'', ``dispersion-based robustness'', and "composite robustness'' respectively. We assess the empirical performance of these robustness formulations in terms of a fixed budget and a fixed target analysis, from which we find that "mini-max robustness'' is the most practical formulation w.r.t.~the associated computational cost.
Show less- All authors
- Ullah, S.; Wang, H.; Menzel, S.; Sendhoff, B.; Bäck, T.H.W.
- Editor(s)
- Rudolph, G.; Kononova, A.V.; Aguirre, H.; Kerschke, P.; Ochoa, G.; Tušar, T.
- Date
- 2022-08-14
- Title of host publication
- Lecture notes in computer science (LNCS)
- Pages
- 63 - 75
- ISBN (print)
- 9783031147135
- ISBN (electronic)
- 9783031147142
Publication Series
- Name
- 13398
Funding
- Sponsorship
- Horizon 2020(H2020)
- Grant number
- 766186